Agents amplify LLMs’ power — Agents act as extensions that enhance LLMs by allowing them to interact with real-world tools and iterate to produce the most accurate responses or actions possible. However, the broader the domain and possibilities for operation, the harder it is to maintain consistency. The advice here is to start with a narrow scope or attract use cases and users that allow you to quickly explore imperfections and iterate on solutions.
Artificial Intelligence is transforming industries at a breakneck pace, and the entrepreneurs driving this innovation are at the forefront of this revolution. From groundbreaking applications to ethical considerations, these visionaries are shaping the future of AI. What does it take to innovate in such a rapidly evolving field, and how are these entrepreneurs using AI to solve real-world problems? As a part of this series, I had the pleasure of interviewing Everton Cherman.
Everton Cherman, CTO and Co-founder of Birdie.ai, is redefining how businesses understand their customers by harnessing over 13 years of machine learning expertise. As Brazil’s youngest Ph.D. in Computer Science, he has developed a unique AI methodology that goes beyond traditional sentiment analysis to reveal the reasons behind customer behavior, enabling companies to proactively prevent churn. Leading a team of innovators at Birdie, Everton is helping major companies like ABInbev and Santander transform scattered customer feedback into actionable insights that drive strategic decisions.
Thank you so much for joining us in this interview series. Before we dive in, our readers would love to learn a bit more about you. Can you share the most interesting story that happened to you since you started your career?
I ended up becoming the youngest PhD “by accident,” but let me start from the beginning:
I started working with Artificial Intelligence almost at the same time I began learning about Computer Science when I entered university in 2005. Motivated by my family’s history of entrepreneurship and by a company founded by two “PhD students” called Google, I initially saw computing and later science, particularly artificial intelligence, as a powerful tool to create products and businesses capable of impacting many lives.
I began following the academic path and published over 50 scientific papers in Artificial Intelligence. However, at a certain point during my master’s/PhD program (I transitioned from a master’s to a direct PhD), I realized I was pursuing a more traditional academic trajectory rather than following the path that originally motivated me: creating impactful tech products.
This realization prompted me to complete my PhD as quickly as possible while simultaneously launching my first startup. The most interesting part is that, seven years after defending my PhD, I stumbled upon an article about record holders for the youngest PhD recipients and discovered that my haste might have earned me the “title” of the youngest PhD holder in Brazil (where I completed my degree).
None of us can achieve success without some help along the way. Is there a particular person you are grateful to for helping you get where you are? Can you share a story about that?
I am grateful to many people, from those who completely shaped the course of my life to those who reinforced a vision I already had. Instead of naming just one, I’ll highlight two key figures:
- My parents — This might sound cliché or even obvious, but understanding their influence has been liberating. The more I understand myself, my values, and motivations, the more I see how deeply they impacted everything I do. My father’s entrepreneurial drive, my mother’s emotional stability and freedom of choice, and their shared resilience shaped me to:
i) have a burning desire to create (my father); ii) embrace freedom and support to choose my destiny (my mother); iii) demonstrate unwavering resilience (both). - Academic mentors — My journey into Artificial Intelligence began with the opportunity to join a scientific research lab (LABI/UNIOESTE) during my undergraduate studies, led by Prof. Huei Diana Lee. Later, during my PhD, I continued my AI studies at LABIC/USP, led by Prof. Maria Carolina Monard, one of the pioneers of Artificial Intelligence in Brazil. Prof. Lee opened me the doors to the academic world and AI, introducing me to Prof. Monard, who guided my advanced studies.
Can you share your favorite “Life Lesson Quote”? How has it been relevant to your life?
“Look at your routine and you will see how far you will go.”
It’s not exactly a quote — I just made it up — but the message holds true. You wouldn’t expect a healthy body and mind without including good meals, exercise, and mental rest in your daily routine, would you? While this principle might seem obvious for physical and mental health, we often overlook its importance in other aspects of our personal and professional lives.
As entrepreneurs or solution creators, how can we craft the best solutions if we aren’t routinely witnessing or listening to our customers’ pain points?
Defining and executing a good routine, rituals, and conscious recurring actions is what sets apart individuals or organizations who achieve their dreams from those who simply “try.”
I take this philosophy so seriously that it inspired the creation of Birdie: a platform designed to unlock and establish routines that connect an entire company to the needs and pain points of its customers. It might sound like a plug, and maybe it is, but it’s the pure truth!
You are a successful business leader. What three personality traits do you think were most instrumental to your success? Can you share a story or example for each?
Translating needs into solutions: My ability to deeply understand someone’s needs — whether they are customers or internal teams — and create practical solutions is a key driver of my daily work. This trait is rooted in empathy and curiosity to fully grasp the other side’s needs and translate them into actionable solutions. For instance, when we started scaling Birdie’s sales, we faced an increasing need to centralize and organize customer feedback from diverse sources (sales calls, product interviews, expert discussions, customer support calls, etc.) to prioritize product and process improvements effectively. Initially, our team tried organizing this information through spreadsheets and task management systems, but the manual effort was overwhelming, and key details about real opportunities for product improvement were often lost.
Although Birdie’s solution addresses this pain point, we are not the ideal target customer for our own product (it’s designed for businesses with thousands or millions of customers, whereas we are a B2B company with dozens of clients). Still, we adapted our solution to meet our internal needs by creating a simple intermediary process: we centralized all conversations in a dedicated Slack channel called “Voice of the Customer.” We added raw, unstructured data to this channel, accessible to everyone in the company. Then, using a ready-made Birdie connector, we imported all these conversations into our system, where AI automatically identified pain points and helped us design initiatives to address them. This shift from a highly manual process to a more automated and insightful approach saved time and allowed us to focus more on understanding and solving customer issues.
Long-term vision with strong short-term execution: This combination is powerful but not trivial. The ability to maintain a clear (and practical) long-term vision while delivering consistent and meaningful short-term results is a major predictor of success or failure for many startups. In AI, this balance is even more critical. The rise of LLMs has opened up a new range of possibilities for creating high-impact products, but achieving ambitious visions doesn’t happen overnight. Often, we must either start with a controlled demo or build a strategic piece of the vision that enables the product to evolve over time. Knowing how to test and iterate strategically and quickly is key to building an ambitious AI vision.
An example of this is a core capability we developed at Birdie: large-scale emergent topic classification. Initially, we relied on an extremely costly algorithm with GPT-4 level accuracy and costs. Our specific vision here was to avoid compromising accuracy during product validation, even if it meant temporarily incurring higher costs. We knew we could drastically reduce costs over time through techniques like training our own models or leveraging the natural evolution and downsizing of LLMs. Long story short, six months after deploying the first model, we had proven the centrality and utility of the capability and had reduced costs by 10,000x compared to GPT-4, while improving accuracy by 15%.
Ok, great. Let’s now shift to the main part of our discussion. Share the story of what inspired you to start working with AI. Was there a particular problem or opportunity that motivated you?
I started in AI (specifically machine learning) because of the opportunity to join the best university lab available in 2005 during my undergraduate studies. Since then, in various moments of my professional journey, I’ve consistently reinforced the belief that AI is one of the primary means to create solutions that were previously deemed impossible. Virtually everything can be disrupted or at least significantly improved with an AI-based solution.
The inspiration for Birdie emerged from a frustrating pattern I kept seeing across businesses. Companies would come to us saying, ‘We have all this customer feedback — social posts, support tickets, reviews, survey responses — but we’re struggling to make sense of it all.’ What struck me was that despite having valuable customer insights at their fingertips, teams were spending countless hours manually sorting through data instead of actually solving customer problems. It reminded me of the early days of search engines, when information existed but wasn’t accessible or actionable. I realized AI could do for customer feedback what Google did for information — transform it from overwhelming data into actionable intelligence. That’s when the idea for Birdie crystallized — not just as another feedback analytics tool but as a way to help businesses truly understand and act on their customers’ voices
Describe a moment when AI achieved something you once thought impossible. What was the breakthrough, and how did it impact your approach going forward?
I was always very skeptical of any solutions related to chatbots. I didn’t believe we had the technology capable of creating conversational experiences beyond being variations of IVRs. Well, it’s obvious now that such capabilities exist, and ChatGPT was the biggest WOW moment in AI for me. We were already testing generative models and were quite impressed by the results, but ChatGPT brought an additional layer of capability that shattered all my skepticism. From that moment, I saw a sea of possibilities opening before me, not only to execute Birdie’s vision faster but also to refine and expand the vision of what could be achieved.
Talk about a challenge you faced when working with AI. How did you overcome it, and what was the outcome?
I’d say the biggest challenge in AI today is going beyond the demo and having a scalable and truly consistent production solution. The issue is that scalability and consistency don’t come overnight but through a lot of iteration and monitoring. One case was the previously mentioned challenge related to large-scale topic classification. We started relatively simply, though costly and with limited scalability, and we had the requirement of not losing accuracy (ideally improving it) while drastically increasing scalability. We achieved this through extensive iteration supported by scalability/cost monitoring and model accuracy tracking. Well-defined monitoring systems were the “secret” to success in this case.
Another case involves our conversational assistant based on agents, which goes beyond a simple RAG (for the more technical folks). The range of questions this agent-based assistant can answer is much broader, but so are the user’s general expectations and the chances of providing incorrect responses. In this case, again, monitoring systems for accuracy, user interactions, and rapid iteration played a crucial role. However, one specific detail made a significant difference here: starting solution iteration with early adopters within the company who are more adept at dealing with LLMs/AI agents. These users not only had more aligned expectations but also actively helped us evolve the product more rapidly, as they understood its potential and were eager to solve issues with our support.
Can you share an example of how your work with AI has had a meaningful impact (on others, on business results, etc.)? What was the situation, and what difference did it make?
One story that really stands out is our work with Mercado Bitcoin, a major cryptocurrency platform. Like many companies, they were drowning in customer feedback — millions of data points scattered across support tickets, social media, and surveys. Their product teams had stopped engaging with customer insights because they couldn’t see how these connected to business metrics. This was exactly the problem we set out to solve.
What fascinated me was watching their transformation. When we implemented Birdie’s AI platform, their teams shifted from spending hours organizing data to actually solving customer problems. The Director of CX told me something that really stuck: ‘We went from having monthly meetings about spreadsheets to having strategic discussions about customer needs.’ That’s when I knew we were onto something powerful — we weren’t just building another analytics tool, we were changing how companies listen to their customers.
This success was also reflected in numbers — an 80% reduction in data analysis and tabulation time as well as a 50% reduction in contact rate for prioritized issues. They were finally able to ‘bring the customer back to the center of executive discussions’ — which is precisely what we set out to achieve with Birdie.
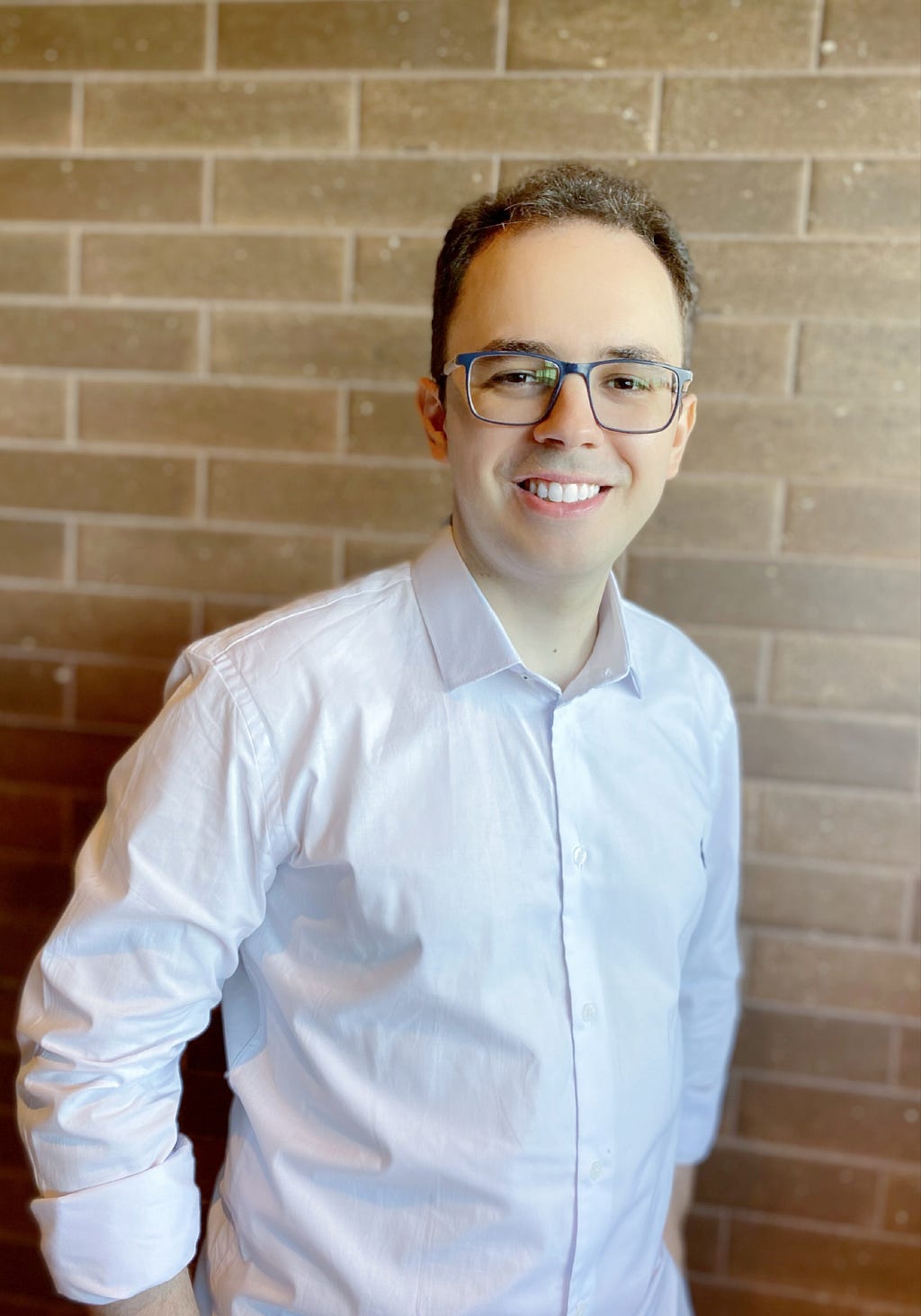
Based on your experience and success, can you please share “Five Things You Need to Know to Help Shape the Future of AI”?
- Start simple first — Begin simply in two ways: with the problem you aim to solve and the solution you initially deploy. Identify recurring problems that are not yet well-resolved but are obviously solvable with AI, even if they seem simple or take little time individually. For instance, it takes only a few seconds for an agent to classify a customer support ticket in a system. Multiply that by thousands of tickets, and you have a significant cost-reduction opportunity for a problem that a simple LLM can address. Why not start there? Some problems may not have straightforward solutions, but have you considered starting with a simple initial implementation that doesn’t fully meet all requirements (especially scalability) at first? The assistant and topic classification examples fit perfectly here.
- 80% is pre-processing and only 20% is actual AI — Invest in robust data engineering, preprocessing, and preparation to achieve the best AI results. In fact, more than 80% of the effort in AI solutions lies in the work before the AI itself. Much of the solution’s foundation is data engineering — from centralizing diverse sources of customer feedback to providing vector-based databases that support better semantic contextualization of tasks executed by AI.
- Agents amplify LLMs’ power — Agents act as extensions that enhance LLMs by allowing them to interact with real-world tools and iterate to produce the most accurate responses or actions possible. However, the broader the domain and possibilities for operation, the harder it is to maintain consistency. The advice here is to start with a narrow scope or attract use cases and users that allow you to quickly explore imperfections and iterate on solutions.
- Your routine is an excellent lab — Test or build tools that support or even automate your routine, whether professional or domestic. This will give you a growing understanding of what is possible with AI. Don’t limit yourself to the basic functionalities of ChatGPT, Gemini, or others. Look for solutions that connect directly to your daily life, from meeting transcription and action item note-taking to automation hubs with API integrations linked to LLMs and communication channel integrations.
- Hype or not hype? — I understand the hype, and in some ways, I’m “hyped” by AI given the possibilities that have emerged. But don’t be naive: demos are demos. Test for yourself whenever possible and see firsthand. Even so, don’t underestimate the power of iteration and time to improve something that’s still incomplete. AI is not just hype or a passing trend for skeptics; it has the potential to significantly change everything we do. However, don’t rely solely on polished, controlled-environment demos.
When you think about the future of AI, what excites you the most, and how do you see your work contributing to that future?
AI will bring a level of automation and productivity that will grant access to solutions and services for many companies and individuals that are currently expensive or scarce. For example, while it might be straightforward for a technical person to make changes to a sales landing page with automation flows, it’s not accessible to everyone. What if you could have your own agent create and modify your landing page and marketing automation based on your instructions? While the best possible approaches will likely still require specialized effort, solutions that effectively meet the basic needs of the vast majority of users can be delivered at a fraction of the cost of hiring a specialist. This more universal access to specific knowledge and services will bring tremendous progress to humanity as a whole.
What advice would you give to other entrepreneurs who want to innovate in AI? Can you share a story from your experience that illustrates your advice?
My most significant piece of advice? Don’t only get caught up in the AI hype cycle. Focus on finding real problems that need solving, even if they seem mundane at first. Looking back, this is what we did at Birdie — we started with fundamental feedback analysis and gradually evolved our capability as we understood our users’ needs better. It’s tempting to try building the most sophisticated AI solution right out of the gate, but I’ve found that starting simple and iterating based on real user feedback leads to much more impactful solutions. The key is to build strong monitoring systems from day one — they’re your eyes and ears into how your AI is actually performing in the real world, not just in controlled demos
Is there a person in the world or in the US with whom you would like to have a private breakfast or lunch, and why? He or she might just see this, especially if we tag them. 🙂
Someone I would definitely want to have coffee with is João Moura (founder of CrewAI). João has unparalleled energy and is leading the wave of agents globally, in addition to being Brazilian! 😀 I’d love to hear firsthand about his experience building and evolving CrewAI. BTW, it’s an excellent framework for experimenting with and creating agents. 😉
How can our readers further follow your work online?
You can visit Birdie’s website, where I keep jotting down my ideas (https://birdie.ai), or you can also reach out to me on my LinkedIn (https://www.linkedin.com/in/everton-cherman/) as well as Birdie’s LinkedIn (https://www.linkedin.com/company/usebirdie/ )
Thank you so much for joining us. This conversation was very inspiring, and we wish you continued success in your important work.
Everton Cherman Of Birdie.ai On Pushing the Boundaries of AI was originally published in Authority Magazine on Medium, where people are continuing the conversation by highlighting and responding to this story.