C-Suite Perspectives On AI: Clare Walsh Of Institute of Analytics On Where to Use AI and Where to Rely Only on Humans
An Interview With Kieran Powell
When the data set is so large that it defies human inspection, you’re going to have to rely on the machine to find patterns for you. For example, I was working on a project with customer review data for a cosmetic company, and the machine spotted a whole new category of customer — women who buy makeup for their mothers. I would never have thought up that customer segmentation.
As artificial intelligence (AI) continues to advance and integrate into various aspects of business, decision-makers at the highest levels face the complex task of determining where AI can be most effectively utilized and where the human touch remains irreplaceable. This series seeks to explore the nuanced decisions made by C-Suite executives regarding the implementation of AI in their operations. As part of this series, we had the pleasure of interviewing Clare Walsh.
Dr Clare Walsh is the Director of Education at the Institute of Analytics. She worked as a highly published author in the education field before re-training in Computer Science. She now leads the drive to upskill professionals in data analytics and the new digital workplace.
5 tips on when to defer human and AI 5.mp4
Thank you so much for your time! I know that you are a very busy person. Our readers would love to “get to know you” a bit better. Can you tell us a bit about your ‘backstory’ and how you got started?
I always say if I can learn data analytics, anyone can. I worked as a teacher and an author. I spent 15 years in an apprenticeship to learn to write school leaving assessments. I may even have worked on your school exams! I kept waiting for someone to change the exam format from my time at school, which predated social media. Assessment analytics are the key to getting that kind of change, so, I went back to university to learn how to do that. Meanwhile, the world changed and suddenly everyone needs a mid-career upskill to stay ahead of digital transformation. I’m here to help.
It has been said that our mistakes can be our greatest teachers. Can you share a story about the funniest mistake you made when you were first starting? Can you tell us what lesson you learned from that?
That first novel data project I tried was ridiculously ambitious. It was a difficult problem and just getting the data into a shape that I could use it was a challenge. Times have changed and that would be easier today. When I got the data to go through, I got fantastically stable results — too good to be true! I really wanted to hear the story that they numbers were telling me, but when I decided to ‘just check again’ I got a very different story. I was trying to measure complex skills in gameplay. I forgot that the kids were playing a game and weren’t necessarily ‘performing’ for the test. They were destroying cities that they were meant to be managing, not because they had no management skills, but just ‘to see what would happen’. They would challenge their friends to a competitive game, and then clearly dumb down their own performance to let their friends win. I was measuring something reliably, but extrapolating all the wrong conclusions from it. The lesson is that the numbers almost always tell you part of the story.
Are you working on any exciting new projects now? How do you think that will help people?
We worked with several governments last year on AI regulation. People want AI brought under democratic control, for good reasons. One conclusion from those discussions is that there’s a skill missing in the work force around AI Assurance to create a more secure future. Many of the laws that are needed to control the worst forms of AI are already in place through existing laws, and the challenge is more around building a body of professionals who can interpret and act on those laws. At the Institute of Analytics, we are a professional membership body for the data professions, we’ve been developing AI Assurance processes. We’re going to have our first batch of qualified AI Assurance professionals finishing that course later this year. We’re really excited to be working in this field at the birth of a new profession that I know will grow in the coming years.
Thank you for that. Let’s now shift to the central focus of our discussion. In your experience, what have been the most challenging aspects of integrating AI into your business operations, and how have you balanced these with the need to preserve human-centric roles?
Intuitive design has made us a bit lazy. We expect to be able to pick up any tool and use it straight away, with no training. Until very recently, workers needed computer programming and mathematical knowledge to run most complex tools, and they had some idea of the limitations of their work. Today, we’re seeing incredibly user-friendly interfaces, in tools like Generative AI, which can look no different to a search engine, and in point and click software that can run machine learning algorithms. Just because you can use it, that doesn’t mean that you’re doing good work or even staying within the rule of law. All these processes have strengths and weaknesses and ethical considerations.
So, the best teams will be making decisions with a combination of machine and human intelligence. You need to do a SWOT analysis on your human-machine team, so that you all know the limitations and strengths of each. Invest a bit of time learning the regulatory considerations around even simple tools like Generative AI before you break any laws. Make all the human and machine assumptions explicit. Many people would prefer an all or nothing approach — I will do Step 2 and the machine will do Step 2. That complete separation in the delegation of responsibility is a recipe for expensive mistakes.
Can you share a specific instance where AI initially seemed like the optimal solution but ultimately proved less effective than human intervention? What did this experience teach you about the limitations of AI in your field?
I remember working with an insurance company. I explained how image recognition works, and they could immediately see the potential of using image recognition in the case of vehicle claims. They could process images of the damage in real time, to make a rapid decision on the extent of repairs needed. With this diagnosis, they could route the car to the local garage able to repair the car quickly. The customer would be less inconvenienced, and costs may be saved. It was a solid business proposal, but the technology just didn’t support that plan.
Most accidents happen in the dark or in poor weather, like pouring rain. Those conditions also hamper the accuracy of image recognition — like a human, a machine can’t see in the dark that well. There was also an assumption that internal damage could be assessed by external markings. Mechanics will tell you that they can’t diagnose what’s wrong without opening the hood. The main concerns were very human, though. We don’t want to put our neighbors and friends in the middle of a busy road, semi-traumatized and confused by the car accident they just experienced. They shouldn’t have to worry about getting a good photo at that moment. It’s just not safe. So, even when something theoretically solves a problem, we need to really look at the problem from both a machine and human perspective.
How do you navigate the ethical implications of implementing AI in your company, especially concerning potential job displacement and ensuring ethical AI usage?
One of the fundamentals of getting AI ethics right is that you have to understand the technology and the social circumstances in the culture and society that nurtured that technology. Pre 2016, IT specialists had almost no ethics training, but most data scientists today have less than 5 years’ experience, and so they have had compulsory ethics training, at least in the UK. We need the rest of the workforce to engage in these questions, now, too.
For example, the duty to use data when it will improve outcomes is a big one. Some older staff just don’t understand how fundamentally things are changing. Traditionally, ‘using data’ means taking a decision based on intuition or feel, and then looking at the sales figures a year or so late to see if it was a good decision. We need to start looking at the data before we take the decision. It may not make a huge difference to practice, but it’s often just enough to give you a competitive edge. And CEOs in 2024 should refuse to make decisions based on a bar chart on a presentation slide! Dynamic interactive dashboards allow the most senior members of staff to easily inspect the whole data set and identify and pick the areas to focus on where there are threats and opportunities. It takes 4 hours and the right tool to learn to build an interactive version. Upskilling is hard, but being left behind technically is also hard. You have to choose your ‘hard’.
So, people who are afraid to make that shift are at risk of being displaced, and small steps today will build up into a big difference 4 years from now. We’re not in the same world today that we were in when manufacturing got offshored in the 1990s, though. We’re not really looking at whole industries, or even whole jobs disappearing. AI automates roles within jobs, not the whole job. For example, radiologists were warned that AI was going to replace them all back in 2019. Today, we don’t have a machine that can replace a radiologist. Instead, we have a global shortage of radiologists because once these experts started to introduce AI insights, they became the ‘super-diagnosers’ of the health world, and demand for their skills soared. Professions like the care industry and religious work are often cited as clearly not under threat from AI, but I’ve advised care home managers on data driven efficiencies and have helped one religious group better understand the experience of dying.
For HR professionals reading, I would urge them to look at the benefits of retaining and upskilling existing staff. In the finance industries, for example, according to one study, although it cost £31,800 on average per person to upskill, the same companies were spending £80,900 on fire and rehire approaches. They also retained important company knowledge to make better human-machine decisions.
Could you describe a successful instance in your company where AI and human skills were synergistically combined to achieve a result that neither could have accomplished alone?
The thing about machines is that they can often tell you what is wrong, but not why. I think the easiest example that many people can relate to is the work I do on assessment analytics. We run the performance data from completed tests through complex analytics after every major test. The machine can help answer the question at the top of our minds: Did we do a good job of asking the right questions? The truth is that test writers never do a perfect job, and behavior to subvert the process of trying to measure genuine ability — like guessing — are common. They all leave data trails.
The machine can locate problems and give some insight into the nature of the problem. But then an assessment expert needs to review it and use all their experience of test design to identify a possible cause. Writing a test brings in so many socio-cultural considerations that the machines just can’t capture, for example, perhaps the test designer mentioned grandmothers or cats in the question. We know that kids who have been recently bereaved will fall apart the moment we remind them of their loss in a high stress moment like a school exam. Getting the machines to moderate the humans, and the humans to moderate the machines produces the optimal environment to get the best result possible.
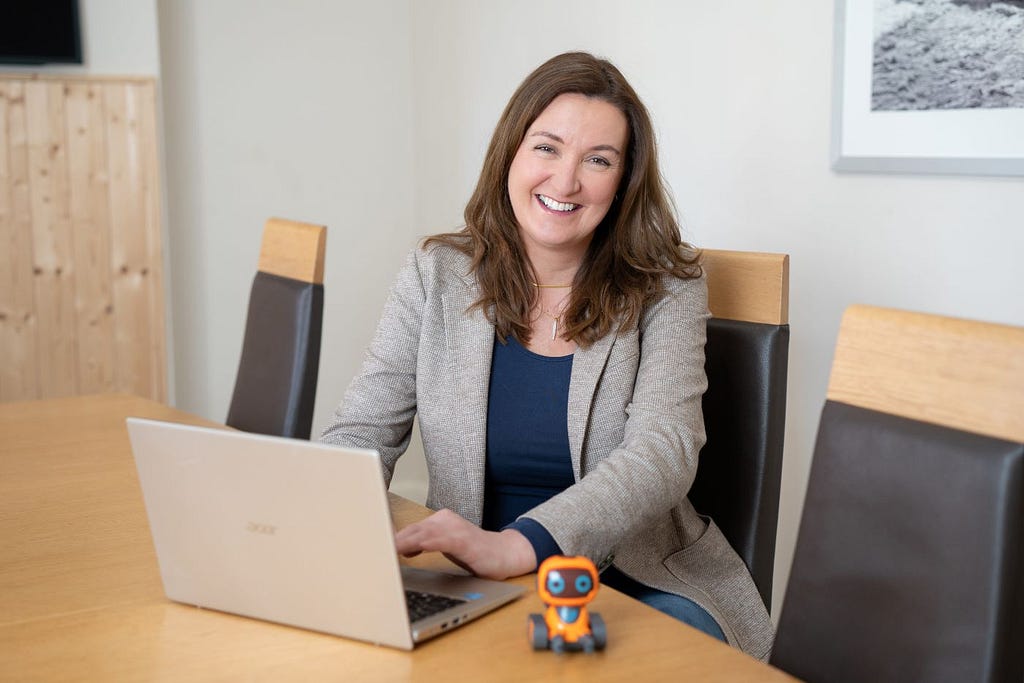
Based on your experience and success, what are the “5 Things To Keep in Mind When Deciding Where to Use AI and Where to Rely Only on Humans, and Why?” How have these 5 things impacted your work or your career?
1 . When the data set is so large that it defies human inspection, you’re going to have to rely on the machine to find patterns for you. For example, I was working on a project with customer review data for a cosmetic company, and the machine spotted a whole new category of customer — women who buy makeup for their mothers. I would never have thought up that customer segmentation.
2 . When the decision is more of a one-off, or is nuanced, then you probably need to defer to a human. The legal profession has a word for this — mitigating circumstances. If you suspect that mitigating circumstances might be involved, put the human in charge. Sometimes people just need to be seen.
3 . When you need a decision made consistently and in a reliable way time and time again, delegate the main authority to the machines. I have a lot of experience measuring how judgments differ between experts, and even the same expert might disagree with their own choices an hour or two later. I know the experience of marking 100 averagely written assignments changes a person! Some get annoyed and become harsher as the process goes on, others just become empathetic to the suffering of their fellow humans and get more and more lenient. So, assume that the either you need to defer the decision to a machine, or at the very least, run some analytics over the human decisions to check how badly they fluctuate. This is true with assessing insurance claims, identifying investment opportunities — everything!
4 . When control is essential, you may want to delegate the task to the humans. On the one hand, relying on things like human data entry is clearly less accurate than automating that kind of process. But bear in mind that with most modern analytics, you are always working with probability. Even when a machine is 95% accurate, which is impressive, it means that once in every twenty cases, things are going wrong. Don’t imagine either that those errors are ‘just a bit off’. They could be spectacularly wrong with AI because do not mimic human reasoning. So, if control is important, make sure you have set up a pipeline for reporting errors, and someone is tasked with reviewing and feeding back into the process. Error is unavoidable, so you need a plan to deal with it.
5. If ownership matters, then think carefully about the role machines play in your process. We rely on complex supply chains in our field and at each stage, there will be nuances in terms of rights and duties. People often argue that copyright is a complex and nuanced thing. In the world of creative arts, there are grey lines, but, in my field, data analytics, the rules are very clear. If I don’t have a checked box giving me consent to load data into an algorithm, I can’t load it. Algorithmic engorgement is becoming more common. This is the legal judgment that a company did not have the legal right to use data and must delete the machine they spent years training. And you do not own all those images you’re creating with Generative AI. Under the contract that you signed when you agreed to the terms of use, the company that trained the machine owns it all. So, if legal ownership is an issue, ask a lot of questions before you get a machine to do the work for you.
This was very inspiring. Thank you so much for joining us!
C-Suite Perspectives On AI: Clare Walsh Of Institute of Analytics On Where to Use AI and Where to… was originally published in Authority Magazine on Medium, where people are continuing the conversation by highlighting and responding to this story.