The cost of AI will go down with time. Early adoption is the key to competitive advantage.
Artificial Intelligence is transforming industries at a breakneck pace, and the entrepreneurs driving this innovation are at the forefront of this revolution. From groundbreaking applications to ethical considerations, these visionaries are shaping the future of AI. What does it take to innovate in such a rapidly evolving field, and how are these entrepreneurs using AI to solve real-world problems? As a part of this series, I had the pleasure of interviewing Niranjan Krishnan, Head of AI Solutions, FPT Software.
Niranjan Krishnan is a seasoned data and AI leader with two decades of experience delivering on the promise of data. He has led large cross-functional teams and deployed dozens of AI/ML solutions that have improved business performance across industries. Niranjan is the Head of AI Solutions at FPT.
Thank you so much for joining us in this interview series. Before we dive in, our readers would love to learn a bit more about you. Can you tell us a bit about your childhood backstory and how you grew up?
I grew up in India, in the suburbs of Madras now known as Chennai. I had a somewhat typical childhood for the place and time; playing cricket on the streets, flying kites on the terrace, and traveling ten miles to the city was an exciting adventure. I loved numbers and solving problems with math, and I was fascinated by trains. I grew up dreaming I’d be a train driver one day, or “engine driver” as we used to call them. It seemed like the coolest job on the planet. I did not become one in real life, but I did earn a master’s degree in Transportation & Logistics from MIT.
Can you share the most interesting story that happened to you since you began your career?
One story I carry with me is about getting an AI solution approved by the legal team of a 150-year-old company. My team built a suite of sophisticated models for running marketing campaigns and optimizing return on marketing investment (ROMI). We were in a highly regulated industry and the level of diligence we went through was eye-opening in many ways. My team and I were forced to think a few decades into the future and literally “future-proof” what we were building.
We spent a lot of time in the general counsel’s office explaining the workings of AI models and the end-to-end solution. We had to walk through every single variable and transformation used in feature engineering. After going back and forth a bit, we decided to pull back on the complex approach and go for an alternative modeling strategy that was less difficult to explain. That upset my team a bit, but it changed the game dramatically. The input variables were clear, the transformations were comprehensible, the model methodology was intuitive enough even for lawyers, the last mile usage was relatable, and the impact on customers was ethical and responsible in a very provable way.
Not only was the simpler solution easier to get approved, but it was also easier to implement without consuming too much storage. The best part was that it was not too far off when it came to predictive accuracy. We rolled it out to run campaigns and ended up improving their efficiency by 60%. We were able to generate more leads for the same budget without compromising on quality.
I carry this mantra with me: Simplicity pays, especially in a complex area like AI.
None of us are able to achieve success without some help along the way. Is there a particular person who you are grateful towards who helped get you to where you are? Can you share a story about that?
The list is way too long and I’m afraid I may miss someone and cause offence. So, I will mention someone from whom I learned how to think early on, especially about how important it is to connect the work you do with ground realities and what matters: Professor Srinivasa Rao from my undergraduate thesis days.
I was designing a complex bridge structure with steel and concrete, all focused on the analysis, design, and esthetics. I had very little clue how much steel, concrete, and labor cost in the market, and was blindsided by the economic aspects of the work I was doing. Not only did Prof. Rao help broaden my perspective and put me on the right track, but he also wrote a glowing recommendation. I’m forever grateful for his generosity. I still carry the lesson I learned about understanding what matters at the end of the day and staying focused on it. This is especially applicable to AI, where it’s easy to get distracted by technology and methodology and lose sight of effort, costs, and creating real value on the ground while generating ROI.
Can you please give us your favorite “Life Lesson Quote”? Can you share how that was relevant to you in your life?
“No force can stop an idea whose time has come.” Attributed to the French writer Victor Hugo, it’s one of my favorite quotes of all time.
Timing matters, sometimes far more than the idea. This is especially true in the context of innovation. Ideas are valuable, but what is equally important is how ready the ecosystem is to adopt the ideas. The idea of Artificial Intelligence has been around for a long time, but the business environment was not ready for it until recently. If you went about proposed an AI solution to a business problem a few years ago, people would immediately tell you it wouldn’t work and quickly lose interest. The idea would be dead on arrival. AI’s time has finally come. In fact, new ideas are now more likely to gain support if they incorporate AI.
You are a successful business leader. Which three character traits do you think were most instrumental to your success? Can you please share a story or example for each?
- Earn trust.
I’ve seen firsthand that trust plays a crucial role in success, especially in business, and more so in my field of expertise: data and AI. Collaborating with various stakeholders who have differing perspectives is essential. It’s common to encounter skeptics and individuals with strong opinions that may not be grounded in data or reality as well as some with their own set of priorities. One of the first things I do for each partner I work with is understand their priorities, identify where I can help, and deliver a quick win. For instance, e-commerce leaders look for ways to understand user activity on the web and mobile to improve performance. So, I start my engagement with them by generating automated insights on user flows across the product surface and identifying actions that can deliver meaningful improvements in engagement, conversion, and monetization.
2. Start with the end in mind and maintain focus
It may seem like common sense, but this point often gets lost in the day-to-day noise or goes out of focus. Businesses are about creating measurable value for their customers. Their strategic priorities and KPIs are geared towards that goal. I usually operate with that end in mind, especially while building solution innovations. I operate with a vision that is rooted in creating tangible value and use technology as the means to realize that vision. Because curiosity and novelty of new technology often wear out quickly, the attention turns to what we can do with the tech and why it matters. Rather than making it all about technology, it is important to align it with strategic priorities, customer value, and KPIs that matter. I find framing solutions in those terms from the get-go is super helpful in securing buy-ins and investments for the longer term, long after the novelty factor wears out.
3. Invest in people
You can’t do it all alone in business or technology. If you have a big enough vision, you’re going to need people to help you get there from partners to those you lead. People work for people, so I make sure I combine EQ with IQ. I am pragmatic but picky about who I hire on my team. Once the decision is made, I make sure I set them up for success. I use a solid framework for hiring, onboarding and growing people, structuring teams with clear career paths, knowing when to step in to help and when to get out of the way, and putting in place an effective operating model with business functions.
Ok super. Let’s now shift to the main part of our discussion. Share the story of what inspired you to start working with AI. Was there a particular problem or opportunity that motivated you?
Firstly, I enjoy solving problems with math and I do that well, I think. I started my career in decision science, working on mathematical optimization of systems. I set out to build solutions to optimize decision-making in businesses, usually involving revenue, cost, profitability, or risk. As I got deeper into it, it became clear that before you could optimize anything, you need to have a system that can predict the range of outcomes one could expect for any given set of business inputs and decisions. For most businesses, making those predictions was a complex challenge. Either the data is not there, the business is changing too fast, the right methodology is not there, or automated solutions are not in place. There was no good foundation in place to enable decision making at scale, and AI was the best way to create that foundation.
To share a specific example, a marketing team I was working with was running digital ad campaigns with dozens of messages and creatives across multiple channels, markets, and audiences. The goal was to maximize conversions from their available budget and to allocate their spending optimally across their channels and creatives. We were able to build mathematical optimization models for this, but a key prerequisite was accurately predicting click-through rates (CTR) for the ad impressions we served. This led to a significant effort in developing machine learning models to predict CTRs, ensuring they operated at scale, implementing a daily retraining process to adapt to changing trends, and establishing rules for allocating ad spend across various channels and creatives. This marked one of my first deep dives into AI — and I’ve never looked back since.
Describe a moment when AI achieved something you once thought impossible. What was the breakthrough, and how did it impact your approach going forward?
The ability to process unstructured data on an unprecedented scale and generate insights and content from it like a human expert does is something I find revolutionary. I personally believe AI has passed the Turing Test which has been the holy grail since the 1950s. This has opened a whole new world of possibilities, and I find myself spending a lot of energy going after opportunities there.
Organizations have accumulated a lot of institutional knowledge over the decades. This knowledge is often not well organized and is dispersed across multiple sources to be usable. Operators managing or running business processes are inundated with a growing pile of service requests from customers, suppliers, and partners. They need to juggle multiple data sources, spending hours to hunt down information, do analysis, and generate insights needed to complete each service request. They had nowhere to turn to for help, but all that is changing with AI. One of the new areas where I help organizations now is in mobilizing and synthesizing their internal knowledge with AI and deploying solutions that drive tremendous value to their operators on a day-to-day basis.
Talk about a challenge you faced when working with AI. How did you overcome it, and what was the outcome?
The #1 challenge in working with AI is data. The data is rarely ready for AI on Day One. Often, the data is not available — if it is available, it’s not in the shape and form to carry out meaningful analysis, let alone build any kind of AI that you could trust.
Ask anyone who has dipped their feet in AI and they would tell you getting the data ready for AI is usually the first order of business. Often that involves investing in high-fidelity data instrumentation at source, followed by robust pre-processing with quality assurance and governance standards.
To give one example, we wanted to build an AI engine to personalize product recommendations for a consumer finance marketplace. It was a multi-channel platform with customer interactions happening across a range of touchpoints: email, web, mobile web, and mobile app — both iOS and Android. We had lots of data about customers and their activities accumulated over the years. But — there is always a but –those mountains of data we had were far from usable. We had different product managers and marketers owning different parts of the platform, and each of them had instrumented and captured data in their own way. Customer activities were not tracked consistently or even captured sometimes. Forget training any kind of AI, it was hard to tell which products or offers were viewed by which customer and where. You could not even trust a simple report on conversion rates. So, before we could do anything with the data, we had to revisit the basics. We had to re-do the telemetry from scratch — who saw what, who clicked what, standardize definitions, introduce naming conventions, appoint data stewards, and put in place a lightweight but effective data governance process to future-proof things. Cleaning up old data retroactively was way too much effort, some of it even impossible, so we decided to wait for the new data to flow through. With just one month’s worth of new data, we were able to build a powerful AI engine with a suite of models covering all touchpoints. Net-net, we can realize over 20% lift in customer engagement and conversions overall.
Can you share an example of how your work with AI has had a meaningful impact (on others, on business results, etc)? What was the situation, and what difference did it make?
Recently I was working with a major manufacturer running a large customer service operation. It was a high-churn environment given the nature of the industry. Tenured reps keep leaving and new ones keep joining. The business was in a high-growth mode, so additional capacity had to be created to keep up with the demand; it was a struggle to onboard new reps and make them productive. Documentation was scattered all over the place and some of the tribal knowledge was in the heads of a few people. It was hard for anyone new to find the information they need to do their job efficiently. We turned to AI for rescue, and lo and behold, we saw the productivity of new reps jump by 20–25% as if by magic. We organized all the documentation in one place, cleaned it all up with the help of AI, and then built a GenAI Agent on top. The reps were able to reduce all the scrambling, find the info they needed instantly, execute tasks faster, and close more tickets every day.
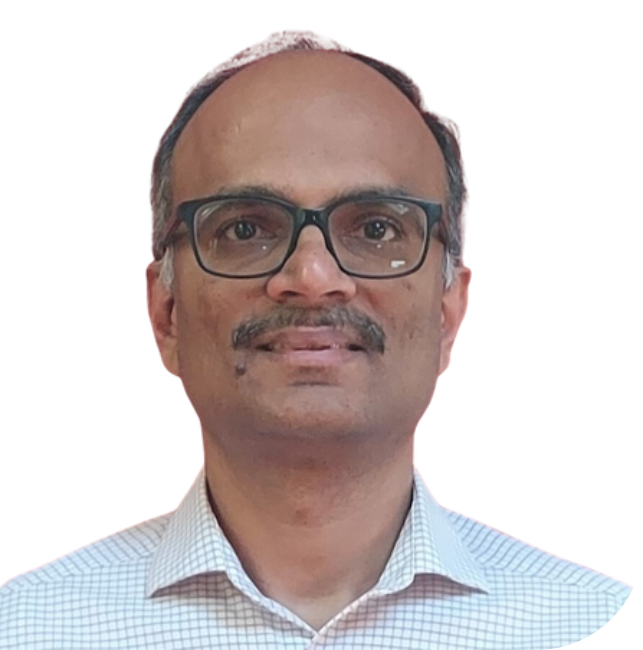
Based on your experience and success, can you please share “Five Things You Need To Know To Help Shape The Future of AI”?
1. AI is real and it’s here to stay.
It may seem like an obvious thing to say, but sometimes it is the obvious that needs to be restated and emphasized. I say this because I get to hear a good dose of cynicism on AI from intelligent individuals. The argument I hear is that there is a long litany of new technologies that got a lot of hype in the last few years but failed to live up to it, sort of fizzled after the sizzle. Think blockchain, metaverse, Web3, NFTs — do we even remember what it means? There is a lurking suspicion that AI too could be heading the same way. The message is worth repeating: AI is real and here for good.
Unlike some other technologies, AI is not the proverbial case of a hammer looking for a nail. It’s more like wheels looking for luggage to latch on. As you know, for a long time, travelers had to physically carry or drag around their boxes and bags. It took a lot of strength and energy. They’re called “luggage” for a reason. Smart minds kept experimenting with the idea of attaching wheels to luggage since the 1800s. But the concept never caught on. Wheeled luggage took off in a big way only in the 1980s, when air travel surged and a pilot thought of adding retractable handles to the boxes. We are at that point right now with AI.
AI can process information on an unprecedented scale and generate results at an unbelievable rate. Predictions, text, code, images, sound, video — AI can whip them out on demand. AI can detect the needle in the haystack, identify connections, and find correlations that would take a long time for humans to detect. After being on the fringes for decades like the wheels for luggage, AI has got its equivalent of retractable handles that make them usable on the ground.
AI can certainly go wrong sometimes and that gives additional ammunition to skeptics and naysayers: Computers cannot think like humans. AI does not have intuition or judgment like real people. AI cannot develop consciousness. While this may be true, it does not take away the fact that AI can be, and is, right most of the time, and it certainly produces output that can be fruitfully used. The pragmatic way to approach AI in my opinion is to focus on the problems it can solve, assess the output it produces, weigh the costs and benefits, and find ways to use that to create value.
2. The secret sauce to AI success is not in the AI. It’s in the data that feeds the AI.
Data is the fuel that really powers AI. The data that organizations accumulate in their systems holds the key to their success with AI. AI models are mathematically complex while AI tools and technologies are technically complex. However, the complexity is getting abstracted away by engaging specialists i.e. experts versed in models and tools, and vendors of products and solutions. What cannot be wished away is the data that feeds the AI. That is specific to your business — you need to manage that actively, with a lot of intentionality.
3. AI comes with risks. But they can be managed with thoughtful strategies.
There is a full menagerie of risks associated with AI that need to be tamed: inference errors, prediction errors, bias, and hallucinations. A popular example till recently was ChatGPT’s inability to correctly count the ‘r’s in “strawberry”. Combined with privacy and security concerns, the risks could be intimidating at first sight. But that should not deter you from adopting AI. Those risks can be managed with targeted actions during the development and deployment of AI. Investing in data quality assurance and responsible AI with input and output guardrails, robust modeling methodologies, stringent security and privacy protocols, ethical standards, and data governance can greatly minimize the risks.
4. The cost of AI will go down with time. Early adoption is the key to competitive advantage.
Like any new technology, AI comes with a price tag. There is a cost to collecting, processing, and storing all the data needed for AI. There is a cost to accessing AI models from third-party vendors and to customizing them for your business and individual use cases. Many of those costs are coming down with time, including storage and computing are coming down with new hardware hitting the market. Costs associated with third-party models are decreasing with more competition. It’s becoming easier for organizations to kick the tires and take AI out for a spin. Plus, AI is fast becoming a table stakes capability that organizations need to have. Early adoption is going to be key. Whoever makes AI work for their business sooner is going to have a clear competitive advantage. Waiting for the technology to fully mature and for costs to come down can leave organizations behind their proactive competitors while early adoption can help enterprises build the AI muscle soon and create sustained competitive advantage.
5. Find problems worth solving. And don’t be surprised if they are boring.
We saw earlier that the data you have is the secret sauce to success to AI. However, the extent of the success is driven by what problems you solve with that data using AI. The high-value use cases are not necessarily the most glamorous ones. Often, they could be tedious but critical tasks that need to get done. Here are a few examples: Analysts finding missing or misplaced signatures in dozens of documents; Inspectors navigating complex hard-hat shop floor conditions to manually examine machines and fixtures to detect defects; customer service agents on a live call with an irate customer or responding to an email, trying to pull up information from multiple screens, matching them, answering questions, and copy-pasting information in multiple systems; technicians trying to locate information in a sea of ancient manuals to fix old machines; accounts payable specialists extracting and reconciling information in multiple bills, invoices, and contracts or agreements to approve payment.
When you think about the future of AI, what excites you the most, and how do you see your work contributing to that future?
I believe we’re just getting started with AI and it’s an exciting time to be in the middle of all the action. I see two distinct streams of activity. One is creating the operating system for AI with the hardware and foundational layers. The second is creating business applications that deliver real value to end users. I see much of my work on the latter part: honing in on things that really matter, building transformational roadmaps packed with problems that are worth solving, solving those problems efficiently and effectively with AI, driving adoption of AI solutions on the ground with win-win proposition for all stakeholders, shaping an organizational culture around AI, creating lasting value for all stakeholders, and realizing the transformation envisioned upfront in way that everyone can see, feel and measure.
What advice would you give to other entrepreneurs who want to innovate in AI? Can you share a story from your experience that illustrates your advice?
I would just riff off the point I made earlier: Find problems worth solving. And don’t be surprised if they are boring.
The big challenge for entrepreneurs is getting the attention of customers and investors. That means leading with an immediate or impactful problem to solve with the ideal outcome including both. AI technologies are improving at a breakneck speed. Tools are getting commoditized and proliferating rapidly as we speak. What gives you the edge when you’re innovating is going after real problems that connect with users and their day-to-day. That requires understanding the customers and their business at a deep level. The use case examples I mentioned earlier are from my own experience. Discussions with customers would often start on a very lofty note like optimizing manufacturing and supply chain operations but would take a different direction on the shop floor or operating center.
There’s a story I once heard that I continue to carry with me.
An appliance manufacturer started seeing a big demand for their washing machines in a particular market in India. The products were flying off the shelves without much pushing. So, the manufacturer decided to go all in and make the most of the momentum. They improved the technology, built an even better model, and started marketing it actively. The product and the messaging ticked all the boxes; the new model was fully automatic, had digital displays, was energy-efficient and needed less power, washed better than before, consumed less water, had more capacity, and so on. Despite the price point also being compelling, the new model did not quite take off like the old one, which continued to sell well like before. The manufacturer decided to dig deeper and sent someone to meet the customers. Soon enough, they found out what was happening. The new model was a front- loading machine while the older one was top-loading. Customers were not using the washers for making laundry but for making lassi (smoothie).
Is there a person in the world, or in the US with whom you would like to have a private breakfast or lunch, and why? He or she might just see this, especially if we tag them. 🙂
Neal Stephenson, he is one of my favorite authors. Cryptonomicon is one of my all-time favorite novels.
How can our readers further follow your work online?
LinkedIn is the best place to follow me: https://www.linkedin.com/in/niranjankrishnan/
Thank you so much for joining us. This was very inspirational, and we wish you continued success in your important work.
Niranjan Krishnan Of FPT Software On Pushing the Boundaries of AI was originally published in Authority Magazine on Medium, where people are continuing the conversation by highlighting and responding to this story.