C-Suite Perspectives On AI: Hamid Tabatabaie Of CodaMetrix On Where to Use AI and Where to Rely Only on Humans
An Interview With Kieran Powell
AI will continue to rely on human medical coders as healthcare continues to advance. There needs to be a continuous feedback loop that requires experienced medical coders who will, over time, inform and improve the quality of automation.
As artificial intelligence (AI) continues to advance and integrate into various aspects of business, decision-makers at the highest levels face the complex task of determining where AI can be most effectively utilized and where the human touch remains irreplaceable. This series seeks to explore the nuanced decisions made by C-Suite executives regarding the implementation of AI in their operations. As part of this series, we had the pleasure of interviewing Hamid Tabatabaie.
Mr. Hamid Tabatabaie serves as chief executive officer and president at CodaMetrix. He has been at the forefront of innovation in medical informatics for over 30 years. His vision, leadership, experience, and insight have helped launch and grow several successful startups in the Healthcare Information Technology (HIT) field. Prior to joining the company, he was the founder and served as chief executive officer at lifeIMAGE, the most utilized cloud-based service for the exchange of diagnostic imaging information. Prior to that, he was the first chief executive officer at AMICAS and took the company from concept to a leader in image management within four years.
Thank you so much for your time! I know that you are a very busy person. Our readers would love to “get to know you” a bit better. Can you tell us a bit about your ‘backstory’ and how you got started?
I moved to Boston as a young man to pursue an engineering degree. I was part of the first generation of students enrolled in the six-year engineering and medical program at a local university. For the first two years, we studied a mix of biological and engineering sciences. Third year, we started to do rounds inside hospitals shadowing doctors as they saw patients. I wasn’t prepared for the profound impact it would have on me. I found myself emotionally invested in patients to the point that I no longer saw myself becoming a physician. However, I knew I wanted to apply my engineering skills to the healthcare world, such that the results of my contributions could improve patient outcomes.
After graduation, I went to work for a large hardware company that served hospitals and health centers. Back then, there wasn’t a notion of an electronic health record, but there was this idea of “best of breed.” Hospitals would buy a pharmacy system and a lab system and use various techniques to connect their information, including re-entry of data. My job was to help hospitals’ IT staff and their respective vendors’ software developers to use our technologies to connect their systems.
Relatively early in my career, I became an entrepreneur and started to launch startups. At one of my early startups, we invented the idea of sharing medical images across wide distances. I’ll never forget a letter I received from a woman whose child had been misdiagnosed with a terminal illness. Using our system, she was able to share imaging information with doctors at Boston Children’s who had a very different take on the diagnosis. They airlifted the baby to their facility, and by the time the mother was writing the letter to me, the child had recovered. I continue to have the privilege of waking up each day determined to work with teams that use technology to make a difference in the way healthcare is delivered.
It has been said that our mistakes can be our greatest teachers. Can you share a story about the funniest mistake you made when you were first starting? Can you tell us what lesson you learned from that?
Back in 1986, there was no email per se, but I worked for a company with an internal email capability. I had just returned from a meeting where I had somewhat boldly suggested that the company launch a fully funded healthcare division. The idea was shut down, saying, “We don’t verticalize.” In frustration, I wrote an elaborate email to the CEO of this huge company. I went to show it to my colleague in the next cubicle. As we were discussing my ideas, I mistakenly pressed “send.”
I got in my car and sped a distance to the CEO’s office to ask his assistant to please delete the message. As I was making the request, the CEO overheard us — he had just finished reading the email. A few days later, the CEO approved expanding into a healthcare division.
What I learned from that experience is that if you have a great idea that’s well-supported and well-articulated, don’t keep it to yourself. Make the case. And make it more than once if that’s what’s required.
Are you working on any exciting new projects now? How do you think that will help people?
In medicine, practitioners primarily use two types of medical codes: codes that describe what tests or procedures were performed and codes that reveal what diagnosis is suspected or represent the current clinical findings. The combination of these two broad categories can result in millions of code sets describing patients’ encounters with healthcare providers. Today, manually and at great cost, either the doctors or professional medical coders enter the code sets into the system after patients’ visits to facilitate insurance payments but not necessarily to inform future care. This is why coding data, known as “claims data,” may be good enough for reimbursement, but insufficient to rely on for clinical insights.
The project we’re working on at CodaMetrix — apart from using sophisticated AI to offer automation at a time of a critical labor shortage in healthcare — is turning notoriously unreliable medical codes into highly dependable clinical information. Our success will allow physicians to use these codes to make better clinical decisions on a timely basis. We’re automating and tagging coding data in a way that speeds up and improves the claims process while making the data buried in the electronic record more accessible, searchable, and useful for clinical purposes. It’s incredibly exciting.
Thank you for that. Let’s now shift to the central focus of our discussion. In your experience, what have been the most challenging aspects of integrating AI into your business operations, and how have you balanced these with the need to preserve human-centric roles?
Years ago, IBM Watson was advertised as the answer to a lot of things, particularly in healthcare. They intimated that Watson would be able to answer questions about how to treat cancer in a certain patient and so forth. While I applaud them for the optimistic view they were chasing, you could equate it to their attempt at boiling the ocean.
In reality, you really need to send your machine and AI system to medical school for every specialty. An AI system needs to learn radiology from radiology experts and surgery from surgical experts and so it goes for every other specialty and subspecialty. AI requires a significant corpus of data to train models, and these must be handpicked by human beings.
Right now, generative AI is the dominant technology story. You can go on the Internet and ask unbelievably detailed questions and get good answers. OpenAI spent tens of billions of dollars training their ChatGPT models to browse much of the data from the web and learn how to provide good answers to nearly any question. But we are not close to being able to do that in healthcare. It’s too specialized, and results can be life threatening. Human beings must create the ground-truth data on which machines get trained for healthcare.
AI continues to benefit from a “human in the loop” approach where people “annotate” and tag the data and create the ground-truth. As our population grows, so does the volume of records that need to be coded. AI can help discern which tasks can be automated to free up human coders’ time to work on more complicated cases and relieve doctors of their administrative burden to focus more time at the bedside. AI will help coder training to be more accurate and efficient to deal with increasing volume of complex cases. One of our core beliefs at CodaMetrix is that “human in the loop” must be at the core of everything we do.
Can you share a specific instance where AI initially seemed like the optimal solution but ultimately proved less effective than human intervention? What did this experience teach you about the limitations of AI in your field?
Bias isn’t just racial, ethnic or gender-based. There’s also algorithmic bias that can impact a healthcare practice that’s utilizing AI. That’s why we test every customer’s data against our models. If there are outliers, we train our models to understand those outliers for every unique customer. We do that all the way at the individual physician level.
How do you navigate the ethical implications of implementing AI in your company, especially ensuring ethical AI usage.
Like most tools, AI can be used for both positive and negative intents. On the negative side, AI can potentially be used to generate codes that result in higher reimbursement, whether or not the higher valued codes are justified and legitimate. AI can also be used to “game” the reimbursement system and avoid payment denials from the insurance company, Medicare, Medicaid, or even the self-paying individuals or companies. The appropriate intent of using AI is to help providers get to the right result, and to reduce the administrative burden on people that provide cases. Our slogan is Code Less, Care More. On the payer side, the appropriate outcome is to use AI to safely and reliably adjudicate claims to be paid automatically instead of absorbing the cost of auditing billions of claims per year.
We navigate this ethical question with a pretty black and white approach. A) We don’t produce codes that are not backed by clear evidence in patient records, B) We provide a detailed audit trail of the evidence on which the predicted code sets are based, C) We also utilize people and models to continuously test customers’ documentation, and to validate the output of our AI models, and D) We make immediate adjustments to automated results that are of low quality or are ambiguous. Our customers have real-time access to analytics and tools that will alert them to any possible misbehavior and will be able to stop the behavior within minutes of an alert.
Could you describe a successful instance in your company where AI and human skills were synergistically combined to achieve a result that neither could have accomplished alone?
Every time I see a doctor, they’re focused on the computer for most of the visit. That’s because they’re searching for clinical information in the electronic health record during their limited time with the patient. CodaMetrix is using AI to change that by synthesizing patient records into codes to help clinicians gain more timely access to relevant and important information.
If providers can clinically rely on medical codes in a patient’s chart, they can focus time on the patient and discuss care decisions. In other examples, if pharmaceutical companies can rely on codes, they can be used to help enroll patients into clinical trials that meet specific criteria to bring a breakthrough treatment to market. If a surgeon can rely on the codes, they can use them to submit information to a national surgical registry that keeps track of how a certain knee replacement works or which heart surgery technique has a lower risk of infection for a particular patient.
AI in healthcare isn’t about replacing jobs. It’s about helping overworked medical professionals do a better job.
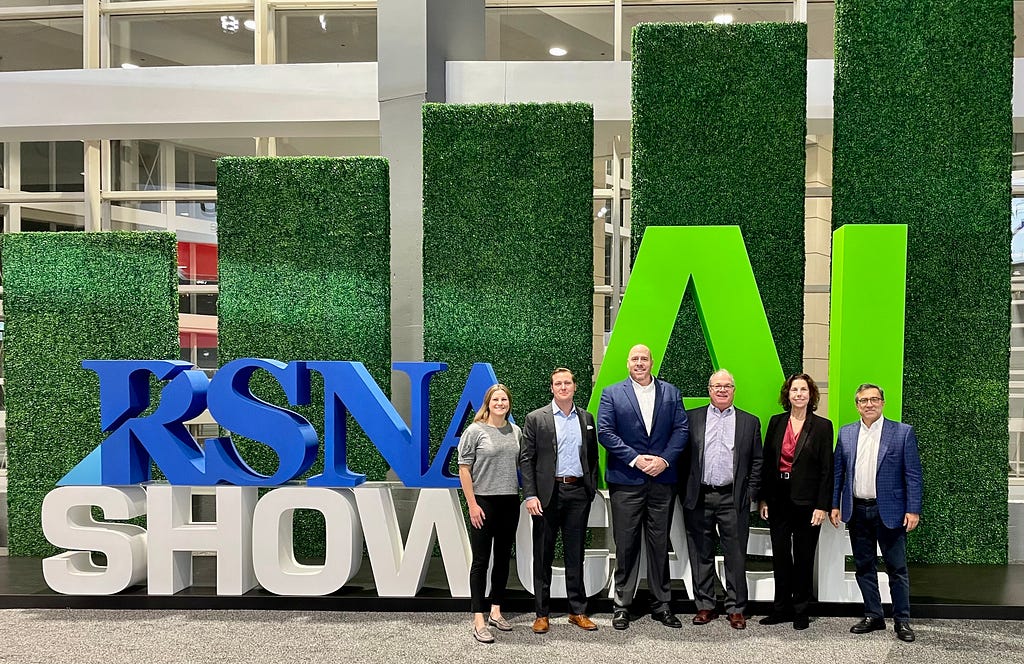
Based on your experience and success, what are the “5 Things To Keep in Mind When Deciding Where to Use AI and Where to Rely Only on Humans, and Why?” How have these 5 things impacted your work or your career?
- AI will continue to rely on human medical coders as healthcare continues to advance. There needs to be a continuous feedback loop that requires experienced medical coders who will, over time, inform and improve the quality of automation.
- There is a gap between clinical processes and administrative processes and their respective data. Health systems are optimized by tuning their clinical systems, processes, and information flow. Traditionally, the administrative side of healthcare has relied on lower cost people and offshoring services to manually deal with the gap that exists between the clinically tuned information and the information needed to process reimbursement needs and other administrative functions. Consequently, there are a glut of point-to-point solutions that serve a narrow purpose, such as reducing denials or measuring infection rates that aren’t captured in a particular system because of a data gap. We believe AI is the connective tissue between the clinical and administrative side. If AI is employed appropriately, it will drive significantly more insights from the patient care process that can serve a lot of use cases without needing complicated, expensive, and hard to maintain point-to-point solutions.
- Providers should determine quality. We build an incredible amount of configurability in our systems so human beings can set boundaries. Giving configurability to the providers to decide on the quality is essential. AI should serve the doctors — not the other way around.
- Stay current. It’s critically important and often no easy task to keep up with the latest medical science and terminologies. Application of new practices in medicine happens every day and we keep training our AI models to adapt. For instance, before COVID, there were no codes for it. We had to quickly adjust to meaningfully apply those codes. Then as COVID mutations dragged on, it had lots of different permutations. Is this long COVID? Is this the viral strain that gives you upper respiratory problems? We tracked those changes and made real-time enhancements.
- Change is hard. Systemwide transformation is difficult, and human beings can naturally resist change. AI is often seen as a shiny new object. It’s incumbent upon us to build tools that make adoption easier, and safer, while making change management tools part of the system. Appropriate and efficient use of AI often requires iterative adoption — and not an all-or-nothing approach.
This was very inspiring. Thank you so much for joining us!
About The Interviewer: Kieran Powell is the EVP of Channel V Media a New York City Public Relations agency with a global network of agency partners in over 30 countries. Kieran has advised more than 150 companies in the Technology, B2B, Retail and Financial sectors. Prior to taking over business operations at Channel V Media, Kieran held roles at Merrill Lynch, PwC and Ernst & Young. Get in touch with Kieran to discuss how marketing and public relations can be leveraged to achieve concrete business goals.
C-Suite Perspectives On AI: Hamid Tabatabaie Of CodaMetrix On Where to Use AI and Where to Rely… was originally published in Authority Magazine on Medium, where people are continuing the conversation by highlighting and responding to this story.